Osteoporosis is a chronic condition of reduced bone strength that is under-recognised and under-treated,1 and can lead to preventable minimal trauma fractures (MTFs). Nguyen et al2 found that only 20% of women postmenopausal women who had a fracture were on specific anti-osteoporotic therapy. Their article suggests ‘most high-risk individuals (possibly 80%) are still not identified and thus not treated’. In Australia, approximately 50% of women and 25% of men have MTFs across their lifetime,3 with an estimated cost of $2.75 billion in 2012.4 Dual-energy X-ray absorptiometry (DEXA) is available to screen for bone mineral density (BMD), and is supported by the national health insurance provider, Medicare Benefits Schedule (MBS), for population screening from ≥70 years of age or following MTFs.5 Underuse of screening has been found to be a problem, particularly for males and rural patients.6
Osteoporosis management options include lifestyle factors (eg adequate vitamin D, calcium, exercise) and medication. In women at high risk, bisphosphonates have been found to prevent vertebral fracture (number needed to treat [NNT] = 20) and hip fracture (NNT = 22).7 Knowing this, and given the availability of Pharmaceutical Benefits Scheme (PBS) subsidies for patients diagnosed with osteoporosis, it is surprising that many patients receive no treatment.8
In Australia, general practice is the hub of chronic disease care for an increasingly ageing population with multiple acute and chronic health conditions. Electronic medical records (EMRs) in general practice documents this patient care and contains a wealth of information that could be useful in primary care research without the need for additional input (ie time, money).9 The use of EMR data overcomes the issue of recall bias,10 and has the potential to provide insights into primary care practice.9 While the EMR is not designed for public health data analysis, it has the potential to efficiently capture real-world prescribing behaviours.11
The aim of this study was to identify rates of osteoporosis diagnosis and treatment in regional Australia using EMR data for all patients aged ≥70 years within participating general practices.
Methods
The study involved an audit, using an adaption of the Canning Data Extraction tool, of an existing general practice EMR database that was previously established (2011) to explore rates of anticholinergic medication use in primary care.12 Ethics approval was obtained from the University of Notre Dame Australia’s Human Research Ethics Committee (reference number 015104S). Written consent was obtained from the 11 practices involved, which were classified as rural, remote and metropolitan areas (RRMA) 2 or 3 within regional New South Wales.
Data were extracted for active patients seen at least three times in the two years prior. Variables collected included patient age, gender, clinical diagnoses and current medication prescriptions. No assessment of quality-of-life variables that may have had an impact on treatment rates was made.
The database included 4853 patients aged ≥65 years; however, this study focused on the EMR data of 3535 patients with aged-based eligibility for subsidised screening and treatment (aged ≥70 years). Descriptive statistics were used for age and gender distribution, and to determine the prevalence of osteoporosis diagnosis and treatment.
Osteoporosis was identified by a manual review of EMR data from the structured (95%) and unstructured (5%) diagnostic field entries, and from bone-active prescriptions (Table 1). The data required recoding of diagnoses prior to analysing the prevalence of osteoporosis and fracture, and bone‑active medication prescription. Fractures and each diagnosis were coded as a dichotomous (‘Yes’ or ‘No’) variable using information from the structured and free-text entries. From the prescription field and free text within the diagnosis field, recorded prescribed medications were reviewed in the same way and coded as a dichotomous (‘Yes’ or ‘No’) variable for any anti-osteoporosis treatment. These were then classified as bone-active medication for raloxifene, strontium, denosumab, or the bisphosphonates alendronate, risedronate, etidronate, clodronate or zoledronate, whether alone or in combination with calcium and/or vitamin D. Teriparatide is not recorded in general practice EMR prescription data. Treatment with bone-active medication was imputed for patients whose diagnosis field included unstructured data that indicated osteoporosis treatment, despite the absence of a bone-active medication recorded in the prescription field. Diagnosis of osteoporosis was imputed for patients whose prescription field included bone-active medication, but there was no relevant diagnosis in the EMR diagnostic item field. The variable ‘osteoporosis identified’ included patients with either recorded or imputed osteoporosis diagnosis.
Binary logistic regression analyses using generalised linear mixed models were conducted for predictors (ie gender, age and fracture history, with practice ID as a random effect) associated with diagnosis and treatment of osteoporosis. All analyses were undertaken using SPSS (Version 23), where a P value of <0.05 was considered significant.
Table 1. Examples of text in EMR diagnostic field to be coded as osteoporosis
Structured
|
Osteoporosis
|
|
Osteoporosis – corticosteroid-induced
|
|
Osteoporosis – no fracture
|
|
Osteoporosis – preventive care
|
|
Osteoporosis with fracture
|
|
Unstructured*
|
|
??OSTEOPOROSIS
|
OSTEOPORISIS HIP - NOT BACK
|
20% T11 LOSS OF HEIGHT
|
Osteoporosis – No fracture BMD – 3.52
|
ACTONEL FOR ?OESTEOPOROSIS
|
Osteoprosis # L4 @ CTscan 9/2011
|
Admitted – Aclasta
|
Outpatients – Aclasta
|
L spine T score – -2.5 for follow up
|
T score – 3.0
|
Osteoporosis
|
T9 Fracture, osteoprosis
|
Osteo-prosis BMD T-4.4
|
T9 wedge vertebra-start fosamax
|
Osteopo
|
Xr-Dorsal vert # – start protos
|
*This list of unstructured coding is at it stands in the database, including spelling errors and capitalisations, to demonstrate the issue of inaccuracies in electronic medical records.
|
Results
The database included 4853 patients aged ≥65 years, with 2745 females and 2108 males. Data from patients aged 65–69 years were used in this paper only for comparison of reported prevalence of osteoporosis with other studies (Figure 1). This study focused on patients with age-based eligibility for subsidised osteoporosis screening and treatment, comprising 3535 patients aged ≥70 years, including 2057 females and 1478 males. Females were aged 70–105 years (mean: 79.4; median: 78.0; standard deviation [SD]: 6.9) and males were aged 70–102 years (mean: 78.0; median: 77.0; SD: 6.1).
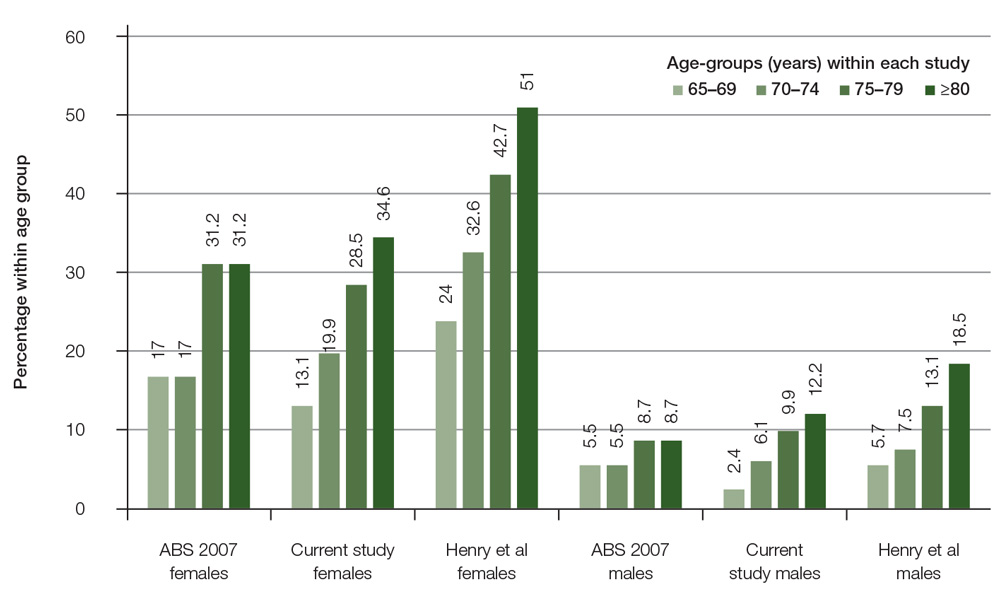
Figure 1. Comparison osteoporosis prevalence by gender and age-groups with other studies
Among patients aged ≥70 years, osteoporosis was identified for 728 patients, 589 females (28.6%) aged 70–99 years (mean: 80.7; SD: 6.5) and 139 males (9.4%) aged 70–92 years (mean: 80.7; SD: 5.5; Table 2). More than 85% of the patients identified as having osteoporosis had a specific diagnosis of osteoporosis in the database. The remaining patients were identified as having osteoporosis by the inclusion of osteoporosis-specific medication in the prescription field of the database.
All predictor variables entered into the model were significantly associated with whether osteoporosis had been identified in patients aged ≥70 years (n = 3535; Table 3). The likelihood of having osteoporosis identified was increased with a record of fracture (odds ratio [OR]: 6.65; 95% confidence interval [CI]: 5.22, 8.47; P <0.001) and with each additional year older (OR: 1.04; 95% CI: 1.02, 1.05; P <0.001). Females were 3.38 times more likely to have had osteoporosis identified than males (95% CI: 2.73, 4.16; P <0.001). The estimate for the random effect covariance for practice ID was 0.124 (95% CI: 0.040, 0.380) and was not significant (P = 0.081).
Of the patients who were identified as having osteoporosis (n = 728), 70.6% were on bone-active medications; 66.2% of male patients and 71.6% of female patients. Although gender and a history of fracture were not significantly associated with whether a patient was prescribed bone-active medication, the likelihood of being prescribed bone-active medication differed by decade of age (Table 4). Compared with the 70–79-year age group, those aged 80–89 years were 1.7 times more likely to be prescribed bone-active medication (95% CI: 1.20, 2.47; P = 0.003). However, those aged ≥90 years were less likely to be prescribed bone-active medication (OR: 0.52; 95% CI: 0.29, 0.93; P = 0.028). The estimate for the random effect covariance for practice ID was 0.625 (95% CI: 0.212, 1.843) and was not significant (P = 0.070). When only decade of age was included in the analysis, those aged 80–89 years were more likely to be prescribed bone-active medication (OR: 1.75; 95% CI: 1.22, 2.50; P = 0.002) and those aged ≥90 years were less likely to be prescribed bone-active medication (OR: 0.53; 95% CI: 0.30, 0.95; P = 0.034).
Table 2. Identification of osteoporosis in patients aged ≥70 years
|
Proportion of patients n (%)
|
|
|
Method of identifying osteoporosis
|
Male (n = 1478)
|
Female (n = 2057)
|
All patients (n = 3535)
|
By diagnosis
|
116 (7.8)
|
505 (24.6)
|
621 (17.6)
|
By osteoporosis-specific treatment
|
23 (1.6)
|
84 (4.1)
|
107 (3.0)
|
Total osteoporosis identified
|
139 (9.4)
|
589 (28.6)
|
728 (20.6)
|
Table 3. Odds ratios for fixed effects: Target – Presence of osteoporosis
Variable
|
Coefficient
|
Standard error
|
Odds ratio
|
95% confidence interval (CI)
|
P value
|
Gender
|
1.217
|
0.107
|
3.38
|
2.73, 4.16
|
<0.001
|
Fracture
|
1.895
|
0.123
|
6.65
|
5.22, 8.47
|
<0.001
|
Age (years)
|
0.035
|
0.007
|
1.04
|
1.02, 1.05
|
<0.001
|
Reference categories: male, no fracture
|
Table 4. Odds ratios for fixed effects: Target – Bone-active medication
Variable
|
Coefficient
|
Standard error
|
Odds ratio
|
95% confidence interval (CI)
|
P value
|
Gender
|
0.308
|
0.308
|
1.36
|
0.984, 2.071
|
0.15
|
Fracture
|
0.180
|
0.192
|
1.20
|
0.820, 1.747
|
0.35
|
Aged 80–89 years
|
0.544
|
0.183
|
1.72
|
1.204, 2.466
|
0.003
|
Aged ≥90 years
|
–0.656
|
0.299
|
0.52
|
0.289, 0.932
|
0.028
|
Reference categories: male, no fracture, aged 70–79 years
|
Discussion
In this study, osteoporosis was identified in almost one in four women (28.6%) and one in 10 (9.4%) men aged ≥70 years seen in general practice. Previous audits of general practice medical records found low rates of identified osteoporosis; for example, Chiang et al13 found that only 12.6% of women and 3.8% of men aged over 59 years had a diagnosis of osteoporosis. The prevalence of osteoporosis reported by the Australian Bureau of Statistics (ABS)14 is similar to the findings in this study, which supports the generalisability of the results.
An epidemiological study that undertook BMD screening on a random sample of community-based men and women aged ≥70 years found that 42.5% of women and 12.9% of men had osteoporosis.15 This may reflect an under-recognition of osteoporosis among community-based elderly people when compared with BMD screening of representative populations. It is also likely that osteoporosis is only being diagnosed when a patient has a fracture. A qualitative study of consumer perspectives of osteoporosis suggested it is seen as a less serious disease than other chronic conditions.16
Similarly, diagnosis of osteoporosis in this study was strongly associated with a recording of fractures in the general practice EMR. Fractures were recorded for 13.7% of women aged ≥70 years in our study, suggesting a possible under-reporting of fractures in this population. For example, the authors of the Australian bone care study17 found that fractures were reported by 29.2% of women aged ≥60 years (n = 69,358), yet, only 47% of reported fractures were also recorded in the corresponding general practice medical files.17 Accurate diagnosis is important for medical treatment, and under-recognition of prevalence will continue to contribute to under-treatment.
The overall treatment rate for patients with osteoporosis aged ≥70 years in this study was 70.6%. An EMR audit in regional Victoria 10 years ago, prior to national subsidies for screening and treatment, found only 58.5% of patients with osteoporosis aged ≥70 years were prescribed anti-resorptive medication.13 A more recent review of medical records using the PEN clinical audit tool (CAT) to extract data relating to risk factors, followed by a manual file audit18 found that 26% of patients (aged 60–95 years) diagnosed with osteoporosis in one practice were not receiving treatment.
In this study, the treatment rate changed with age. Three quarters of patients with osteoporosis aged 80–89 years were treated, compared with only half of the patients aged 90–99 years. Possible reasons for the absence of a recorded current treatment prescription for 29% of patients with osteoporosis include medication side effects, medication contraindications,19 patient awareness, patient choice, GP concern about financial barriers, the salience of osteoporosis and GP clinical judgement of holistic patient needs.20 A patient would need to have a predicted life expectancy of at least three years to achieve a benefit from such treatment.21 In this study, there were no significant differences in treatment rates between genders.
Funding models may also exacerbate diagnostic and treatment gaps. Current MBS funding structures give priority to acute rather than preventive healthcare and care of chronic conditions. While general practice management plans benefits for patients with chronic conditions such as osteoporosis have been funded since 2005,22 the model remains unadjusted for the number or severity of comorbidities affecting each patient, which are more common in the elderly.23
Previous published research has documented the potential to improve treatment rates. A Melbourne-based, pharmacist-led intervention used individual general practice academic detailing to significantly increase treatment of patients with osteoporosis aged ≥50 years from 59% to 70%.24 A larger study in Western Australia used cycles of general practice audit, reflection and review to significantly increase the bone-active medication treatment rates after MTFs from 76% to 86%.5 The Western Australia study also noted that general practice audit and review could increase the rate of BMD screening of patients aged ≥70 years with no fracture history.5 The methodology used in our research to review EMR data in a general practice setting has the potential to provide a framework for future studies to evaluate the success of interventions to improve access for osteoporosis.
Limitations exist in the use of data extracted from EMR in primary care research, despite the use of individualised data extraction tools (DETs). It has been argued that EMRs are a poor source of public health data, as GPs have varying interest and expertise in data management.25 However, although issues such as incomplete and missing data exist,26 these can be addressed by recognising data limitations and adequately scrutinising the results.27 This study found that the EMR diagnosis field in particular needed recoding and imputation prior to analysis (Table 1). The prescriptions listed in the EMR database may not have been recently updated, leading to possible inaccuracies of current scripts.
Despite these limitations, we feel there is considerable scope for the use of DETs in the Australian general practice setting, particularly as a measure of change in practice. This study has added to the body of evidence that supports the use of DETs, as well as to knowledge about osteoporosis in the general practice setting. Refinement of these tools with targeted software development28 may address some of the potential limitations into the future.
Implications for general practice
- Despite the fact that men and women aged ≥70 years are eligible, not all patients receive MBS-subsidised osteoporosis screening and prescribing of active treatments.
- It is likely that MTFs remain under-recorded in general practice records.
- The use of structured, pre-coded diagnosis categories rather than free-text diagnosis entries would facilitate more effective auditing.
- Audits have the potential to identify osteoporosis under-treatment.
Authors
Megan Elliott-Rudder MBBS, FRACGP, PhD, Postdoctoral Researcher, Wagga Wagga Rural Clinical School, School of Medicine Sydney, The University of Notre Dame Australia, NSW
Catherine Harding MBBS, FRACGP, MHPEd, MPH, PhD, Head of School, Wagga Wagga Rural Clinical School, School of Medicine Sydney, The University of Notre Dame Australia, NSW. catherine.harding@nd.edu.au
Joseph McGirr MBBS MHSM, FRACMA, Associate Dean, Wagga Wagga Rural Clinical School, School of Medicine Sydney, The University of Notre Dame Australia, NSW
Alexa Seal BSc (Hons), PhD, Research Assistant, Wagga Wagga Rural Clinical School, School of Medicine Sydney, The University of Notre Dame Australia, NSW
Louis Pilotto BSc, MBBS, PhD, FRACGP, FACRRM, Conjoint Professor, Rural Clinical School, Faculty of Medicine, University of New South Wales, NSW
Competing interest: None.
Provenance and peer review: Not commissioned, externally peer reviewed.