Some of the earliest and most renowned general practice researchers, such as Charles Bridges-Webb, William Pickles and John Fry, began their research by using routinely collected clinical data to answer important primary care epidemiological questions. In the past two decades, with significant developments in electronic healthcare data, there has been growing interest in the research utility of so-called ‘administrative data’, or data collected routinely about whole populations. This paper discusses a specific aspect of research using administrative data: data linkage.
What is data linkage?
Data linkage has been defined as ‘the bringing together from two or more different sources, data that relate to the same individual, family, place or event’.1 The concept of data linkage was first proposed by Dunn in 1946, where he suggested the creation of a ‘book of life’ for each individual from birth to death, incorporating key health and social events.2 This would be a compilation of existing records to create an individual file for use in health service planning, and to confirm the accuracy of data across sources.
Internationally, data linkage systems and facilities exist in relatively few countries, including Canada, England, Scotland, Denmark and the US. The earliest Australian endeavour to link data began in the 1970s in Western Australia and led to the creation of the WA Data Linkage Unit in 1995. In 2006, the Australian Population Health Research Network (PHRN) was created and funding was provided to develop data linkage units in all states and territories to support state, national and cross-jurisdictional data linkage.3
These state-based linkage units are supported through a variety of university and non-government units. These include The University of Western Australia and Curtin University in Western Australia, which support and coordinate the PHRN, The Sax Institute in New South Wales, which provides secure mechanisms for access to data, and The University of Melbourne and BioGrid Australia, which provide data linkage services. The types of dataset that have been linked as part of these national initiatives include births, deaths, perinatal outcomes, hospital admissions, emergency department attendances and cancer registrations. In some states, there are more extensive linked datasets, including mental health, genealogy, education, criminal justice and child protection, and specific research cohorts (Figure 1).
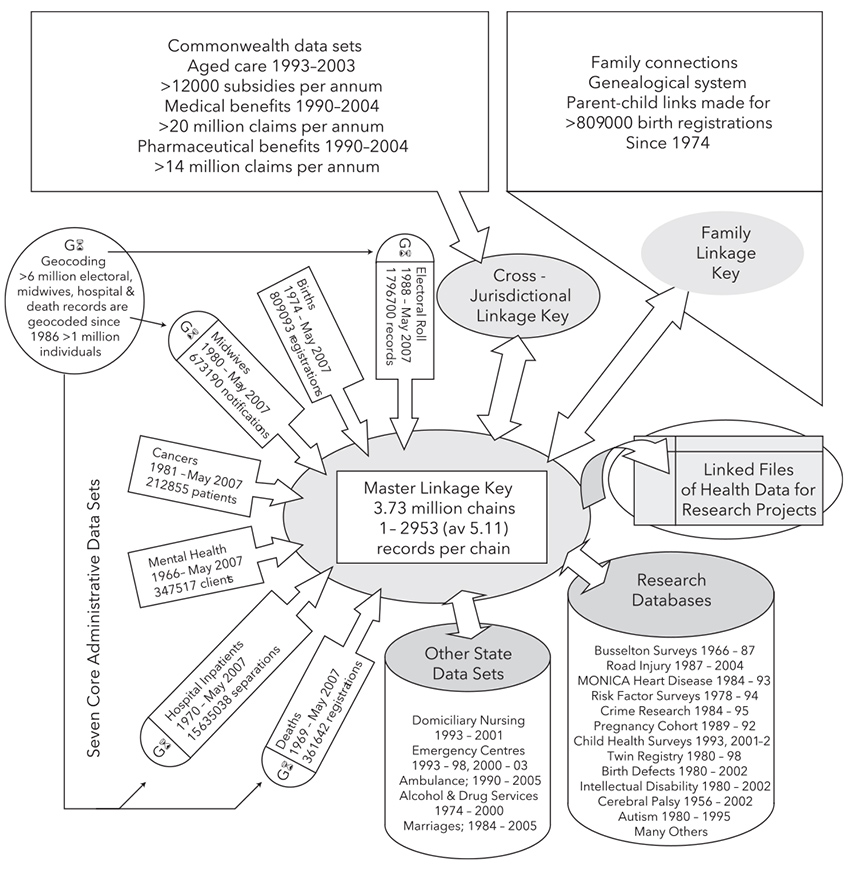
Figure 1. Example of datasets linked in WA Data Linkage system1
Reproduced with permission from Holman CD, Bass AJ, Rosman DL, et al. A decade of data linkage in Western Australia: Strategic design, applications and benefits of the WA data linkage system. Aust Health Rev 2008;32(4):766–77.
How are data linked?
There are two commonly used approaches to linking data:
- Deterministic linkage, where an individual has one or more unique identifiers (eg National Health Service number in the UK) that can be used to allow complete matching between datasets.
- Probabilistic linkage, where unique identifiers across datasets do not exist or use of unique identifiers is restricted (eg Australia). In this case, matching is performed using partially identifying variables that are not unique (eg name, date of birth, address), and a probability calculated that this represents the same person. This is a complex process that involves multiple passes through the dataset to assign weights and likelihood of a true match. Different thresholds of probability of a true match can be pre‑determined to balance precision and sensitivity of the data linkage.4,5
Privacy and ethics
Concerns about privacy are the most significant ethical issue raised by data linkage. In most cases, consent will not have been obtained for use of these routine data for research purposes. Linked data may also be considered potentially re-identifiable data. An important aspect of maintaining privacy is the ‘separation principle’. Identifiable demographic data (eg name, address) are separated from the clinical data prior to linkage by the data linkage unit. This ensures clinical data are not accessible to data linkage officers. The demographic data are compared between datasets to identify records belonging to the same person. A linkage key is created, which allows the identification of records belonging to the same person. This linkage key is then used to identify the clinical records for that person, held by each data custodian, while ensuring the identifiable information remains protected from the researchers (Figure 2).
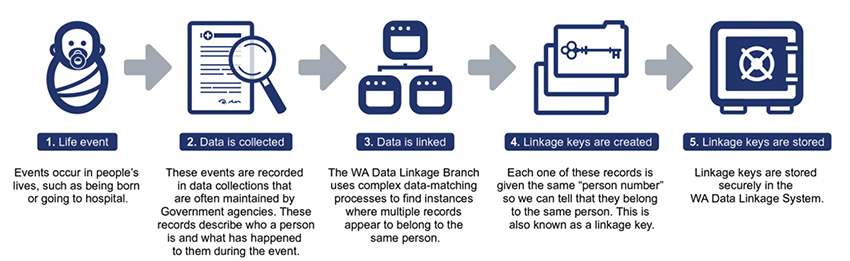
Figure 2. Probabilistic data linkage process5
Reproduced with permission from Data linkage Western Australia. About us. Available at www.datalinkage-wa.org.au/dlb-linkage-extraction-process [Accessed 30 June 2017].
Technologies that generate non‑reversible linkage signatures take privacy a stage further, whereby the signatures or keys are generated before data leave the participating data custodian organisations. Because no identifiable information leaves the contributing organisations, there is no prospect of a breach of privacy during data linkage. In some cases, policy or legal restrictions mean identifiers cannot be released for submission to a linkage unit, making signature-generating linkage technologies the solution to data linkage. However, verifying the quality of data linkage in these instances is very difficult because of the inability to perform human review of medical records. For this reason, identifiable record linkage and privacy-preserving, signature-based linkage both have their place.
Researchers who wish to apply for access to linked data from a data linkage unit must provide a detailed data security plan to the unit and the human research ethics committee approving the study, and obtain approval from the data custodian of each linked dataset. Several guides and checklists have been developed to ensure best practice data security. These guides and checklists cover key aspects of protecting identity, physical and technological security, transport, retention and disposal of data.6,7
Uses of linked data
Linked datasets in Australia have been used for studies of health service outcomes and use, epidemiology and needs analysis. Examples include:
- precise estimates of deep vein thrombosis risk associated with long‑haul flights8
- trends and factors associated with increased use of caesarean section9
- risks of birth defects from different types of assisted reproduction10
- effect of human papillomavirus vaccination on subsequent cervical cancer screening participation rates.11
The majority of such studies have been limited by their access to data relating to services delivered in primary care. As discussed, most of the linked health service datasets relate to care provided in hospitals. The two main potential sources of data relating to activity in Australian general practice are Medicare data (ie Medicare Benefits Schedule [MBS] and Pharmaceutical Benefits Scheme [PBS] billing data) and clinical data extracted from individual general practices.
Linking Medicare data
The first studies to link MBS and PBS data were conducted through the WA Data Linkage Unit in the mid-2000s, and tested the feasibility of cross-jurisdictional data linkage (ie linking federal-held and state-held datasets). This created an additional and significant layer of complexity to research and ethical governance, but the fundamental principles of probabilistic linkage as described above remain the same. These studies were an important proof of concept and led to a number of important findings, including:
- the effect of regular chronic disease care in general practice on reducing mortality and hospital admissions for chronic respiratory disease,12 ischaemic heart disease13 and epilepsy14
- the effect of general practitioner (GP) care on the risk of hospitalisation from use of potentially inappropriate medicines by the elderly in the community15
- the use of general practice services by people with chronic mental health problems.16
Access to linked Medicare data is improving through the PHRN, but it is important to recognise some of the limitations of these data. The vast majority of MBS consultation items provide no information about the reason for the visit; exceptions include, for example, specific items relating to mental health, chronic disease management and health assessments. MBS data are more specific for investigations ordered, although they do not provide information about test results. Perhaps the greatest strength of Medicare data from general practice is the detailed information on prescribing and dispensing provided by the PBS data, although one should recognise that not all drugs prescribed in general practice are on the PBS. These are potentially of significant value for pharmaco-epidemiological research.
General practice clinical data
Several tools exist in Australia that can extract clinical data from general practice electronic medical records. Many of these have been developed primarily to support practice audit and feedback; more recently, these have been used by Primary Health Networks (previously Medicare Locals) to inform health service planning. The Medicine Insight program, led by NPS MedicineWise (www.nps.org.au/health-professionals/medicineinsight), extracts clinical data from more than 500 Australian general practices in a secure way that enables linkage to other datasets. Our research group is currently leading a proof-of-principle study to link Medicine Insight data with hospital data from the Victorian Comprehensive Cancer Centre partners to examine aspects of general practice care across the cancer continuum. The data extraction technology is also being used to investigate mechanisms to identify patients who have a high risk of hospital presentation.17
Internationally, the most well-developed clinical general practice dataset is the UK’s Clinical Practice Research Datalink (CPRD, previously known as the General Practice Research Database [GPRD]), which was first established in 1987 (Figure 3; www.cprd.com/home). This is now linked to several other datasets, including hospital admissions, outpatient and emergency department attendances, diagnostic imaging and cancer registration. The CPRD has resulted in some highly significant research findings, including confirming the safety of mumps, measles and rubella vaccination, informing guidelines on the symptoms of cancer in primary care, and the management of hypertension in patients with diabetes. It is also providing research infrastructure to support the conduct of large-scale trials in primary care.
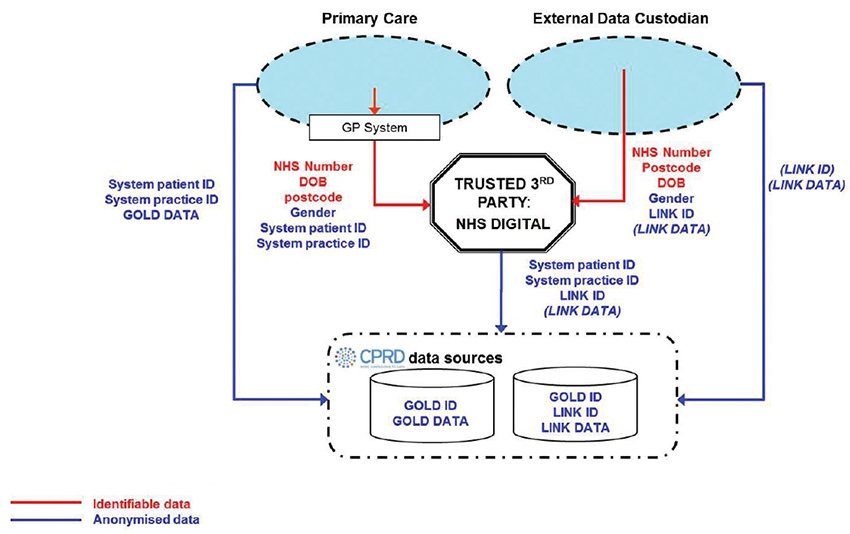
Figure 3. Flow of data from practices and data linkage in the CPRD
Reproduced with permission from Clinical Practice Research Datalink. Home. London CPRD, 2017. Available at www.cprd.com/home [Accessed 28 June 2017].
There are potentially important limitations with routine general practice data.18 The quality of the data is highly dependent on how GPs record data in their electronic medical record. General practice clinical software systems allow data to be entered as coded and ‘free-text’ data. While there have been major developments in natural language processing to support the interpretation of free-text data, most research currently relies on the use of coded data only. When establishing a clinical general practice dataset, significant investment is needed to work with practices to improve the quality of coded data to achieve accurate and complete data. Various approaches exist to validate data; a systematic review of validation studies of diagnoses in the GPRD found a median confirmation rate of 89%, but this varied across studies and diagnostic category.19 GPs may not necessarily see the value of increasing their entry of clinical information as coded data. Free-text entry comes more naturally and is closer to traditional handwritten medical notes. Involvement in quality improvement activities that rely on the use of coded data (eg clinical audits) may help to break down this significant barrier to the creation of high-quality, usable clinical data.
Conclusion
This brief overview of data linkage demonstrates the potentially important contribution this approach can make in health services and epidemiological research. Linking general practice data to other healthcare datasets presents significant opportunities to answer research questions that might not be readily answerable using other research methods. However, it is important to understand the significant challenges relating to data quality, data analysis and research governance to ensure such research is conducted securely and with appropriate interpretation.
Authors
Jon Emery MA, MBBCh, MRCGP, FRACGP, DPhil, Herman Professor of Primary Care Cancer Research, Department of General Practice and Centre for Cancer Research, Victorian Comprehensive Cancer Centre, University of Melbourne, Vic. jon.emery@unimelb.edu.au
Douglas Boyle PGDip IT, BSc, PhD, Director of the HaBIC R2 Unit, University of Melbourne, Vic
Competing interests: None.
Provenance and peer review: Commissioned, externally peer reviewed.